This content is provided in partnership with Tokyo-based startup podcast Disrupting Japan. Please enjoy the podcast and the full transcript of this interview on Disrupting Japan's website!
Japan is lagging behind in AI, but that might not be the case for long.
Today we sit down with Jad Tarifi, current founder of Integral AI and previously, founder of Google’s first Generative AI team, and we talk about some of Japan’s potential advantages in AI, the most likely path to AGI, and how small AI startups can compete against the over-funded AI giants.
It’s a great conversation, and I think you’ll enjoy it.
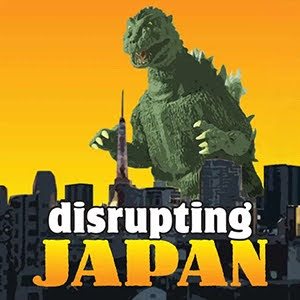
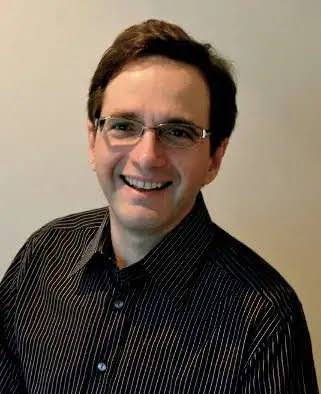
***
Transcript
Welcome to Disrupting Japan, Straight Talk from Japan’s most innovative founders and VCs.
I’m Tim Romero and thanks for joining me.
Japan is lagging behind in AI, but that was not always the case. And it won’t necessarily be the case in the future.
Today we sit down with Jad Tarifi, current founder of Integral AI, and previously founder of Google’s first generative AI team. We talk about his decision to leave Google after over a decade of groundbreaking research to focus on what he sees as a better, faster path to AGI or artificial general intelligence. And then to super intelligence.
It’s a fascinating discussion that begins very practically and gets more and more philosophical as we go on.
We talk about the key role robotics has to play in reaching AGI, how to leverage the overlooked AI development talent here in Japan, how small startups can compete against today’s AI giants, and then how we can live with AI, how to keep our interest aligned.
And at the end, one important thing Elon Musk shows us about our relationship to AI. And I guarantee it’s not what you, and certainly not what Elon thinks it is.But you know, Jad tells that story much better than I can.
So, let’s get right to the interview.
(Part 2 of 4. Continuing from Part 1.)
***
Interview
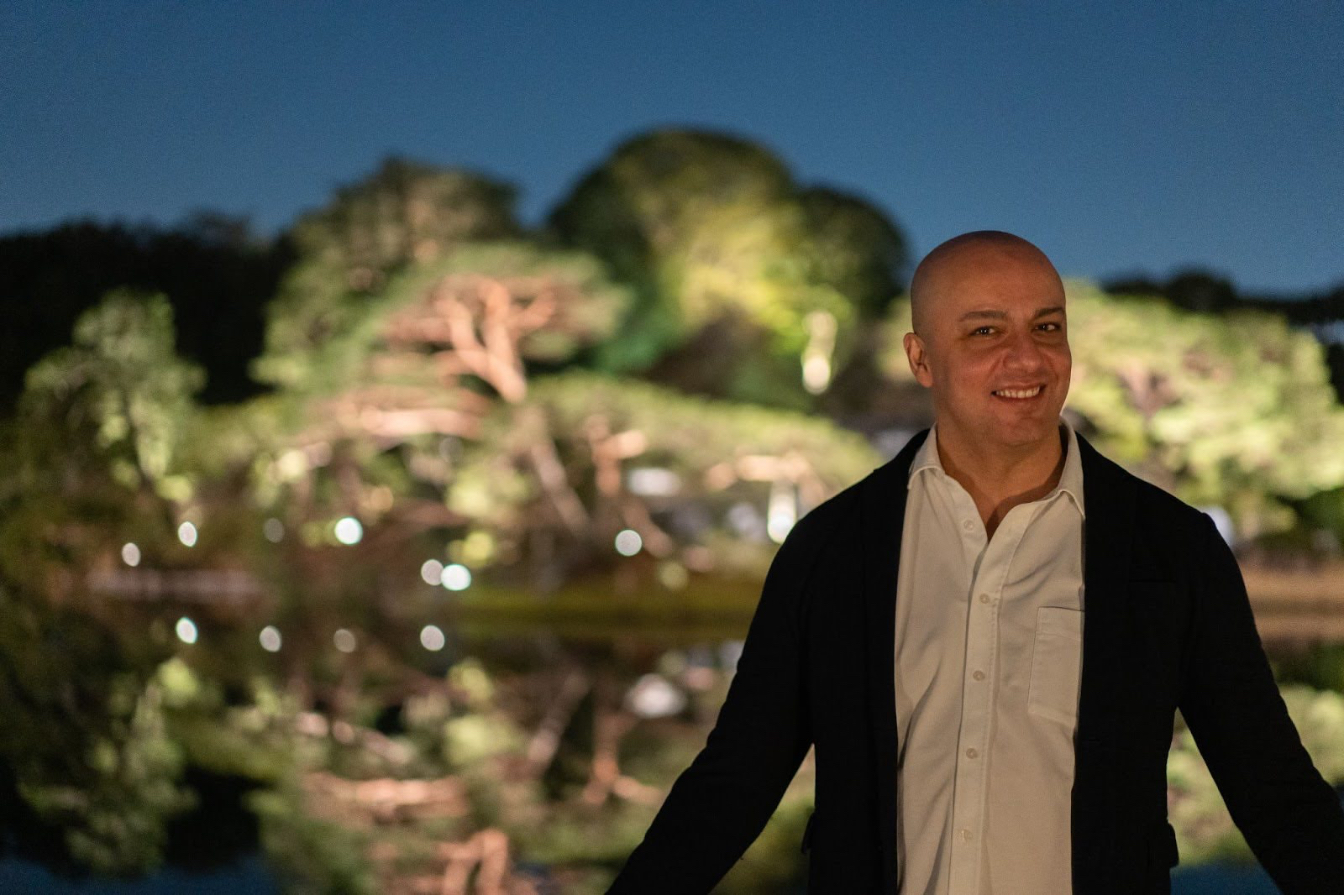
Tim: I want to put a GI to the side for a second. I really want to get back to that, particularly as it applies to robotics. But before that, I want to ask you one question about your corporate structure. So you’re based in San Francisco, but you have offices here in Tokyo, I mean, we’re sitting here in Tokyo, you’ve got engineering teams here in Tokyo, I believe. So why so many companies are saying that there’s this talent shortage in Japan, they’ve got to go to San Francisco to find engineers, but you’re having success right here in Japan. So what are you seeing that so many other startups are missing?
Jad: The first is, well yes, there’s a lot of talented AI researchers in the Bay Area, but the robotics industry is relatively immature in the US compared to Japan. Japan is about 50% of the world’s industrial robot production. So we have a very strong ecosystem on the robotics side based in Tokyo. So our thought was to combine the primary area for AI, which is Silicon Valley and the primary area for robotics, which is Tokyo, Japan. This has been a really good bet because what we found is that there’s a lot of underappreciated engineering talent here. There is a language barrier. So one of the things we got really good at is helping young talented engineers get comfortable in an English speaking environment. We have a lot of best practices to help them kind of adapt to that. And usually it takes about three months to six months for them to get comfortable. The other thing is that we are not a research lab, so we don’t really need too many senior AI researchers exploring different ideas. We have a clear vision about what we want to build. And so it gets easier in the sense that we can afford to hire a lot more junior people. The value of someone with high energy, high integrity, great teamwork is much more than like industry experience for us at this stage.
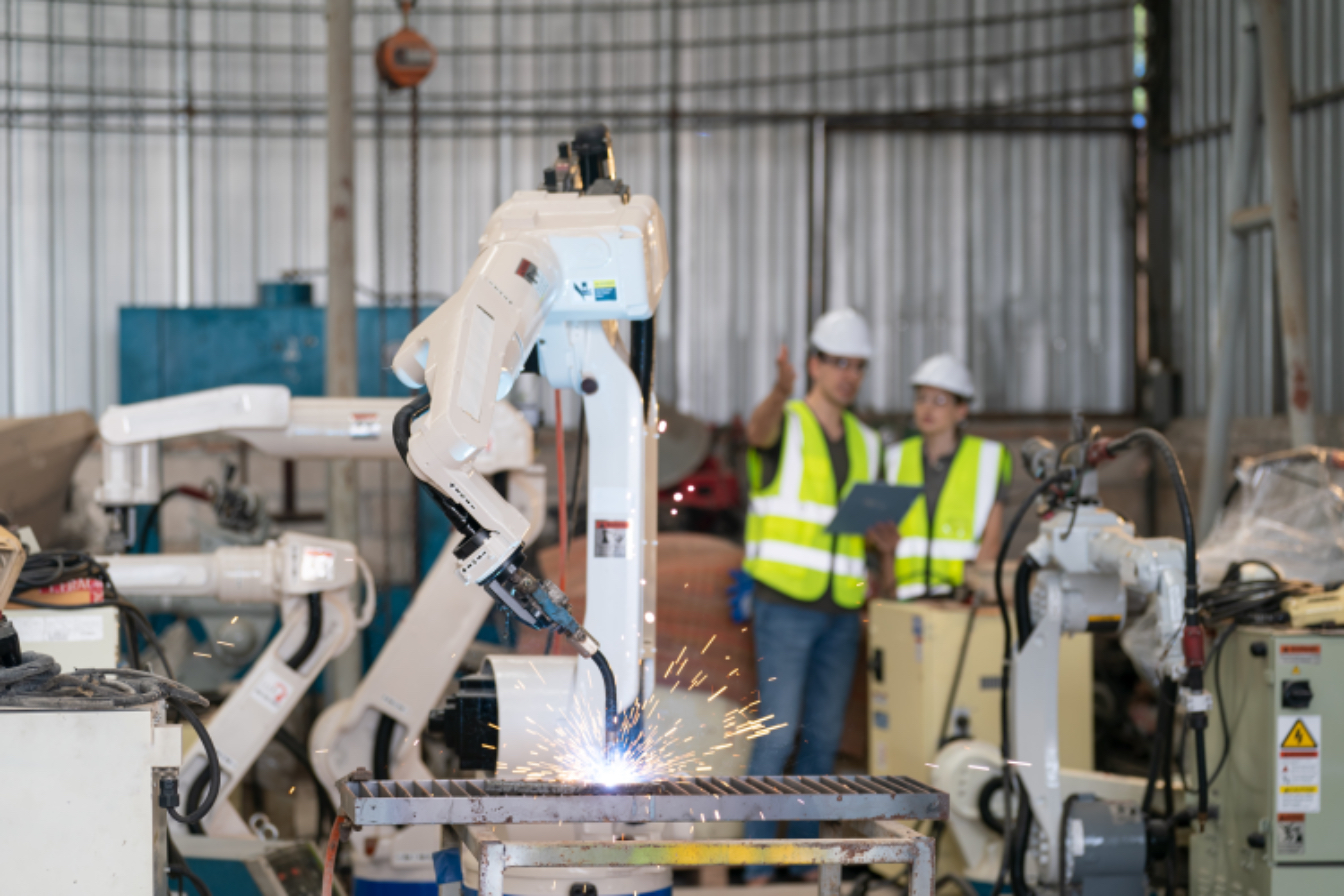
Tim: So, any advice you have to all of those startup founders who think they need to go to the Bay Area to find talent?
Jad: I think to be open-minded is very important. It’s very attractive to say let’s go to the Bay Area. But in fact, a lot of the entrepreneurs I know in the Bay Area would say it’s too expensive in the Bay Area. Talent is too fickle. You hire someone, you train them for a few months and then they go to the next job. A bigger picture. I think we want more diverse opinions to be shaping AGI, we don’t want just one physical location, one monoculture. So I’d like to see that kind of spread out a bit more around the world.
Tim: So Japan is, as you mentioned, traditionally very strong in robotics, but robotics is hard. I mean, most startups fail, but I mean really almost all robotics startups fail. Why is it so hard to make money here?
Jad: This is a great question. I think we can spend an entire interview just on this question first. There’s this tendency when you are in a small startup to be overwhelmed by the robotics stack. There’s too many things, too many difficult things that you need to solve. And so what you end up doing is saying, I’m going to build this one product and let’s see robotic picking, and then I’m going to collaborate with this robot arm company and then collaborate with a system integrator and then sell to the company, there’s too many players and nobody has the complete ownership of on the product. And when no one has the complete ownership on the product, it’s hard to iterate.
Tim: Right. So you can’t like push something to market, right?
Jad: Yes.
Tim: It’s too much of a consensus building.
Jad: Exactly. So you end up in a state where it is just not favor startups that have to move fast, that that’s a huge bottleneck. And, and, and in order, if you want to own the whole thing, you need a lot of technical expertise and you need a lot of resources, which startups are not known to have. The second…
Tim: But wait, no, let me push back on that because even companies like look at Boston Dynamics. So they’ve got the resources, they’ve got the technical expertise, they have God it seems like unlimited funds, but they just can’t bring a product to market. I mean, they’ve got sales, but it’s all these kind of cool little pilot programs and even they can’t seem to like really be a robotics startup success story al although they have incredible tech.
Jad: I would challenge the incredible tech part. I think their tech is more traditional stuff. And I think they’re also adapting because they’re realizing the traditional control theory approach that they took is not really that scalable, but their demos are cool and impressive and inspiring. Now I like your pushback because you’re needing naturally to my second point. The second major point is, how attractive is it to have an automated solution that is specialized? So if you are a factory assembling phones in China, here’s the unfortunate reality. You can choose to buy a robot or you can choose to hire someone for $10,000 a year. And the metal on the robot itself is already more than $10,000 a year. So customer acquisition is very hard because again, there’s availability of cheap labor. Second, the sales cycle is very long. Sometimes I talk with some big name, very big name companies in Japan and they’re planning a factory. And I say, great, when are we going to go to production? And they say, oh, 2032.
Tim: That makes sense. They’re not going to re-engineer their line every year, right?
Jad: So you’re in the construction business, you’re not really in the technology business. The third component to why startups have failed in robotics is unfortunately the technology wasn’t there yet. You needed to have different technology per use case. So you need to basically get an engineering team, design a solution for every use case. And that adds up to the total cost of the system. But as we enter the generative AI era and AGI, we are going to have one solution that works across a wide variety of problem.
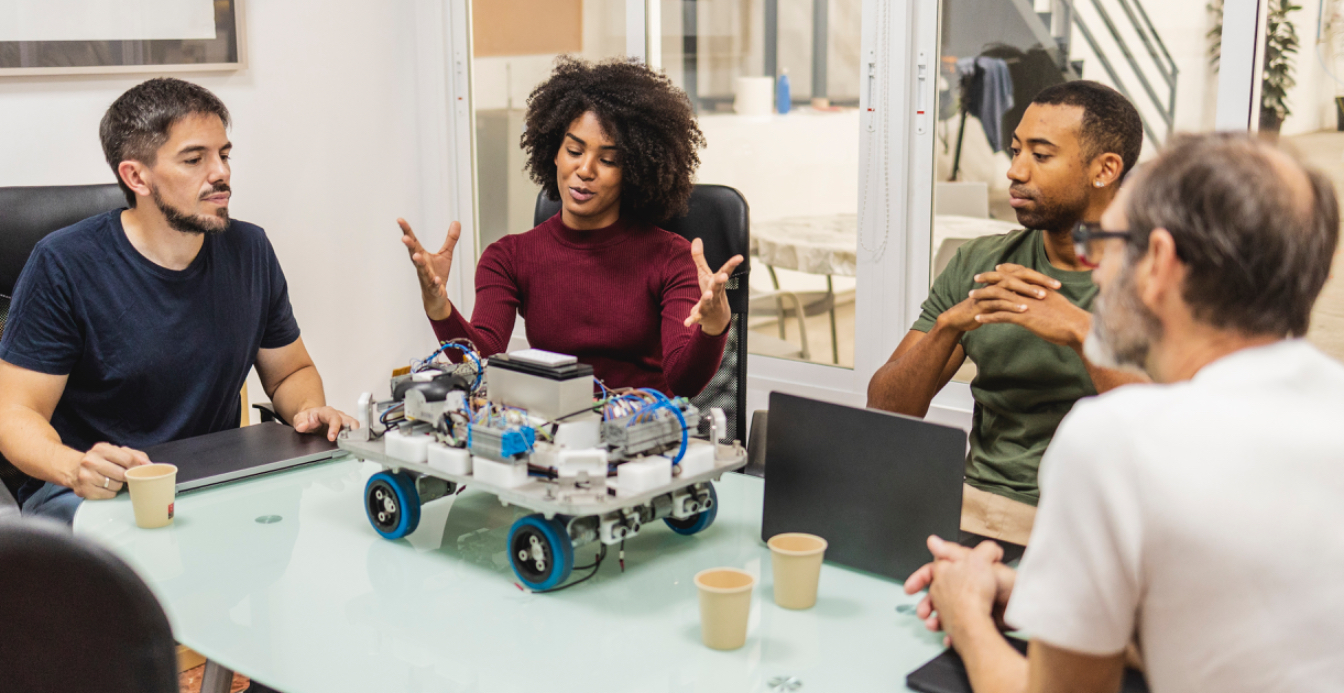
Tim: How universal is robotic training data. If I have data from an auto factory robot and I’ve got data from, I don’t know, Boston Diamonds Atlas and I have data from a robot that makes, makes and serves coffee, how much use is training data from one domain to another?
Jad: This is great question and it’s a common misunderstanding. In the investment community in Silicon Valley ecosystem, they assume that robotics is hard because there’s not enough data and that you need to go out and collect data for every robot environment. And that’s part of the story. For example, why human aids are great because you have one form and then you can collect data for, but actually a lot of how we learn is not through interaction, but in watching other people do something. In fact, what we found is that if you train a model to understand the world, it can apply across different bodies. You do need some interaction with the new body, but that’s just like stretching out before you go to the gym. And actually, it turns out the more the model that does the stretching generalizes well. So, if you trade on 10 types of robot, the 11th robot will be even easier. So we see a world where there’s going to be a whole Cambrian explosion of robot bodies, many, many different robot types doing different things.
Tim: Okay. But that sounds like a really great machine learning AI niche to be focused on right now. But if I could ask you a broader question about AI startups in general. So what are your thoughts, on how smaller AI startups can compete with these firms that are making foundation models like OpenAI, Anthropic, and Meta have unlimited funds, great talent, and without moving into things like hardware strictly within the software space, do you think there is defensible business models within generative AI right now?
Jad: I do. And I think your question can be divided into sub-questions. I’ll first answer how to compete against a larger company and then we’ll discuss the business model because that’s also very interesting. If you are just training a transformer model, I don’t think there’s much hope there because what you’re doing is clear to everyone. But if you do have a technology differentiation in terms of the architecture, the algorithms of what you’re doing, there is a chance. But that’s not enough because everything can be copied eventually. So what’s the startup advantage? It’s always the ability to make decisions quickly, iterate fast, so you have a more ability to explore an uncharted territory. And that becomes very important when you have a platform change. When you have a platform change, there’s a lot of opportunity and the winner is usually the one who can explore that faster.
Tim: To dig down on that, what would be some domains or some, some concrete examples where that might make sense? Because I see so many AI startups and most of them are like, wow, that’s a clever idea. But if that market ever got big enough, one of the big companies would take a small step sideways and take it from you.
Jad: It needs to be a platform change. It cannot be a feature, it has to be a fundamental platform change. That’s one Second, it has to have a strong defensibility to, so there has to be a moat. So right now we’re in the middle of a platform change and one of the platform changes towards LLMs. The classic example there is Open AI. Open AI was much less resourced in every way in terms of data, in terms of talent, in terms of financial resources than Google. And we are seeing right now, Google is struggling, I would say the least compared to Open AI. And this is not the first time. I mean, Google did that to Microsoft. So whenever you have a platform change, you have an opportunity for startups to win purely because they can maneuver faster. The second very important thing is that you have the capacity to build a moat. Clearly to me, in the physical world, there’s a huge moat when it comes to having models that continually grow with time and benefit from Gale on data. So if you have a model that is up to date that a lot of people already use, it already contains so much more information than what you can find publicly or you can train from scratch. Now with traditional generative AI, you can’t do that well because you have this model that you train once and then you run in inference mode. One of the breakthroughs we’ve unlocked in our company is the ability to do continuous training. The model can add new data, can add new experiences and not forget. So, there’s a problem in neural network called catastrophic forgetting. We’ve solved that problem in a clever way. And so this opens up the opportunity for a strong network effect on data.
Tim: Let me flip the question. Do you think there’s defensible value in building these foundation models? GPT, Lama, Claude, they all have different characters when you use them, but honestly the objective performance differences aren’t that obvious. And it’s all Google’s transformer architecture, it’s all open source. There’s not really IP that’s defensible here. So looking at Open AIs $157 billion valuation, safe super intelligence just raised a billion dollar pre-seed round. Is this defensible? I mean long term are these valuations going to be justified not for the technology mind you but for these specific companies or are we going to see lower cost competitors coming to the market?
Jad: So, first I want to make a distinction between the success of any one company versus a technology. The technology itself is world changing and if any one of those companies succeeds and manage to scale it up, then whoever invested in that is going to get the multiples and is going to justify the investment ecosystem. Is there something defensible about building a model from scratch? I would say no. the best it can give you it’s a head start. So even our technology is not transformer based is completely new yet we don’t believe its defensible if that’s the only thing. What’s defensible is always the ecosystem you can build, the relationship with the end user. And there is a bigger point here, this is such an important change for humanity maybe it doesn’t matter if many companies die trying.
Tim: That what I think might be a very likely scenario here, I mean I know its completely different but like the solar industry in the late 1990s, 2000s I mean so much money was lost. Most of those companies went out of business but the technology itself continued to advance and its having a transformative impact on the world. And I’m wondering if we might see the same thing with generative AI with the technology goes on to have a transformative effect and 20 years from now the investment community is looking back going my God what were we thinking.
Jad: I would agree that the vast majority will die but the bigger picture doesn’t matter because the social benefit will be there. And from an investor perspective there’s still some companies that will make it and those will be worth your investment.
(To be continued in Part 3)
In Part 3, we’ll hear about why Integral AI chose Tokyo as its base, the challenges faced by robotics startups, and the business models that allow AI startups to compete with major players.
[ This content is provided in partnership with Tokyo-based startup podcast Disrupting Japan. Please enjoy the podcast and the full transcript of this interview on Disrupting Japan's website! ]
Top photo: Envato
***
Click here for the Japanese version of the article